The RF environment for many advanced RF applications is increasingly congested and possibly contested (see Figure 1). Emulating this environment in field tests can be very costly and, in some cases, is not feasible.

Figure 1 Replicating the “fog of war” of the battlefield in field tests is infeasible or prohibitively expensive.
Information Systems Laboratories’ (ISL’s) RF Digital Engineering family of tools, known as RFView®, can facilitate the life cycle of advanced RF systems—radar, electronic warfare (EW), electronic intelligence (ELINT) and other advanced RF applications—from development through sustainment (see Figure 2). With ISL’s RFView hardware-in-the-loop (HWIL) solution, for the first time, actual flight hardware can be integrated into a high fidelity modeling and simulation (M&S) environment via an RF analog or digital interface.
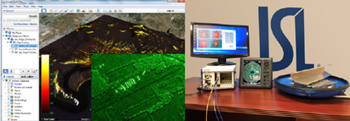
Figure 2 ISL’s RFView HWIL solution enables real-time virtual field testing in environments as complex as a designer’s imagination.

Figure 3 DARPA’s KASSPER project, a predecessor of cognitive radar, provided an impetus for advanced RF M&S and “digital twinning.”
ISL’s technology began with seminal projects at the Defense Advanced Research Projects Agency (DARPA) and the Air Force Research Laboratory (AFRL). In 2000, DARPA/AFRL initiated the Knowledge-Aided Sensor Signal Processing and Expert Reasoning (KASSPER) project,1 a predecessor to what today is called cognitive radar.2–4 Its goal was to create a new generation of “smart” radar capable of adapting to complex environments too stressing for traditional adaptive radar (see Figure 3).
From the start, it was clear that a new generation of high fidelity, physics-based RF M&S tools would be required to develop, evaluate and test the methods developed in the KASSPER project. To fill this gap, ISL was selected to apply the advanced M&S tools it had already begun to develop. Beginning in the early 2010s, AFRL initiated the Cognitive Fully Adaptive Radar (CoFAR) project under the Small Business Innovation Research (SBIR) fund, with a sister project focused on M&S to support the effort. So successful, it resulted in a rare Phase III award focused on transition.5–6 (see Figure 4).

Figure 4 AFRL’s CoFAR project was an SBIR success story.
A fully commercial set of products has since been created that form a fully integrated set of digital engineering tools supporting the whole product lifecycle, from requirements and analysis-of-alternatives, through design/prototyping, test and evaluation, training, mission planning and sustainment (see Figure 5). This environment is ideal for artificial intelligence and machine learning systems, which require voluminous training data and/or environments to ensure robust performance.

Figure 5 ISL’s RFView Digital Engineering tools support the product lifecycle.
References
- J. R. Guerci and E. J. Baranoski, “Knowledge-aided Adaptive Radar at DARPA: An Overview,” Signal Processing Magazine, IEEE, Vol. 23, No. 1, 2006, pp. 41-50.
- S. Haykin, “Cognitive Radar: A Way of the Future,” IEEE Signal Processing Magazine, Vol. 23, No. 1, 2006, pp. 30-40.
- J. R. Guerci, “Cognitive Radar: The Knowledge-Aided Fully Adaptive Approach,” Artech House, 2010.
- J. R. Guerci, “Cognitive Radar: The Next Radar Wave?,” Microwave Journal, Vol. 54, No. 1, January 2011, pp. 22-36.
- D. Metzger, “SBIR Phase III Award Information,” SBIR-STTR, August 2019, Web: www.sbir.gov/sites/all/themes/sbir/dawnbreaker/img/documents/Course9-Tutorial3.pdf.
- “SBIR-STTR Success Story: Information Systems Laboratories Inc.,” SBIR-STTR, November 2018, Web: www.sbir.gov/node/1526807.